Why is my arm a bottle? The absurdity of image recognition systems for people wearing prosthetics.
State of the art image recognition systems often label prosthetics as items such as hairdryers, chairs, and bottles. Together with the Amputee Care Center by Spronken in Genk, Belgium and people wearing prosthetics, we explored the impact of such misrepresentations and reimagined how these systems can be build more inclusively.
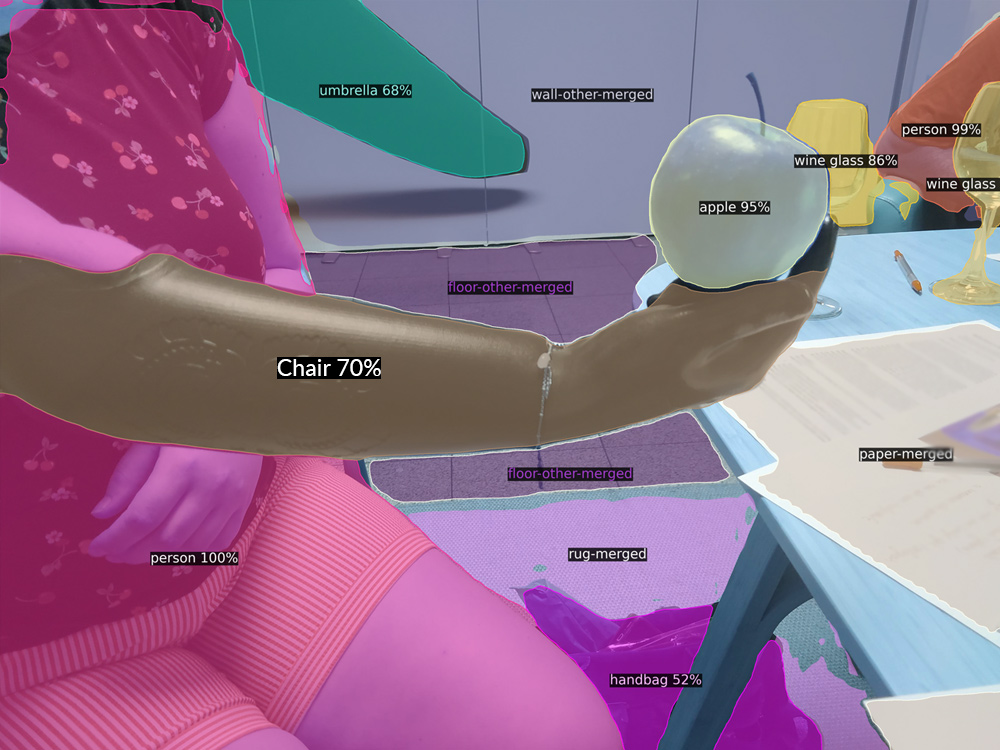
Context
Image detection systems have enormously improved over the last couple of years. State of the art models can recognize people almost flawlessly in general use-cases.
However, not everyone has the same bodies, and it is here that image recognition systems still have a lot to learn. As can be seen in the sections below, state of the art systems have numerous problems with recognizing people wearing prosthetics, often referring to these prosthetics with rather denigrating terms, such as chair or toilet.
This article aims to make data science teams more aware of this bias in image recognition systems and offers insights into making these systems more inclusive of people wearing prosthetics.
Approach
Together with the Amputee Care Center by Spronken in Genk, Belgium, we invited four people with prosthetics, one occupational therapist, and Femke Loisse – the coordinator of the center – to explore the results of a state-of-the-art image recognition system* on prosthetics.
The goal was to better understand in which ways the system interprets their prosthetics, what the impact of these interpretations on the participants can be, and how they would like the system to change to be more inclusive of them.
The results of this exploration are detailed in the following section, after which a conclusion is given on how data scientists can incorporate the feedback of the participants.
This work is seen as an initial exploration of the topic to encourage more inclusive design of image recognition systems for people wearing prosthetics and give practical direction for data scientists to start that journey.
Findings
Absurd labels for prosthetics
We quickly observed that the image recognition system often labels people wearing prosthetics in absurd ways. For example, in the images below, the system names a participant’s prosthetic arm as a bottle.
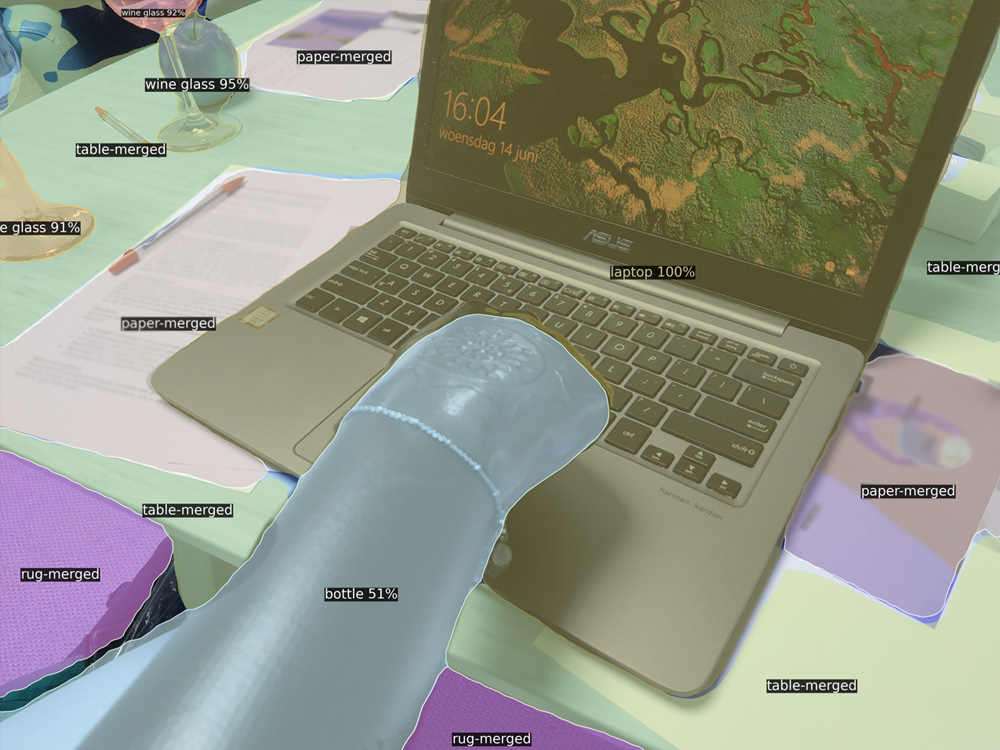
Obviously, “bottle” is a wrong label. But how would a system need to name prosthetics? Are they part of the body and should they simply be part of the label “person”, or should they have their own labels? If they should have their own labels, then how should they be named?
Though there were differences in how participants would like their prosthetics to be named specifically, they all agreed the prosthetics need to be named. It should not simply be part of the label “person” (as in the following image below), but have a label in and of itself. One participants suggested using vernacular names depending on the region and culture.
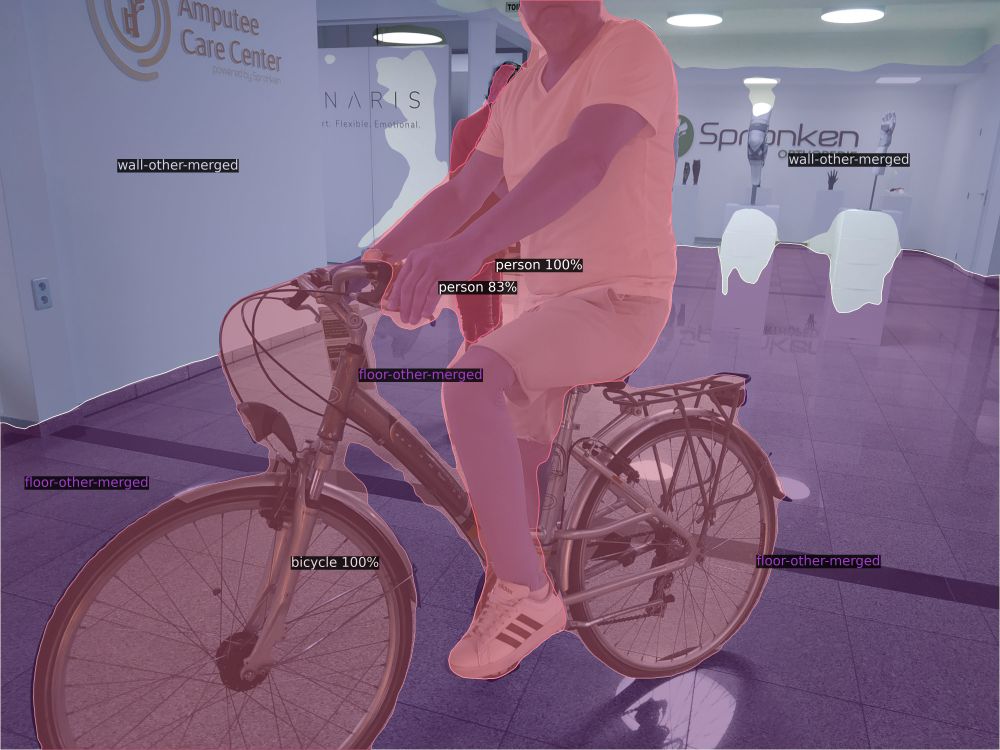
The coordinator of the center also found it strange that the system did not label a prosthetic in and of itself either, as depicted in the two images below. For an important technology like image recognition, she would expect it to know such a basic object.
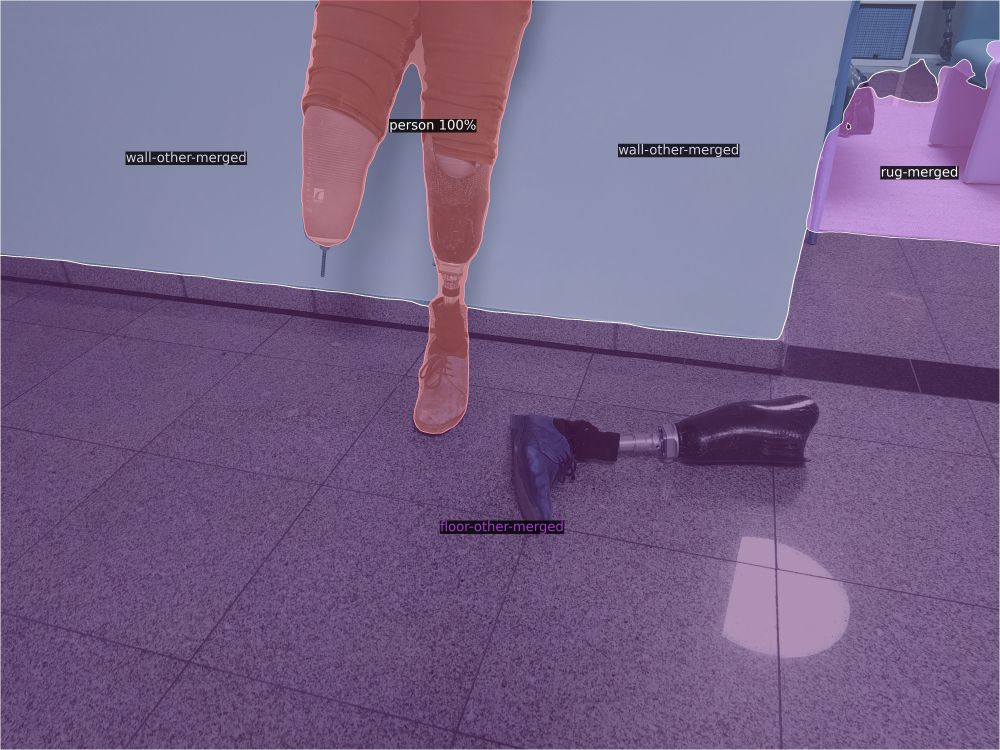
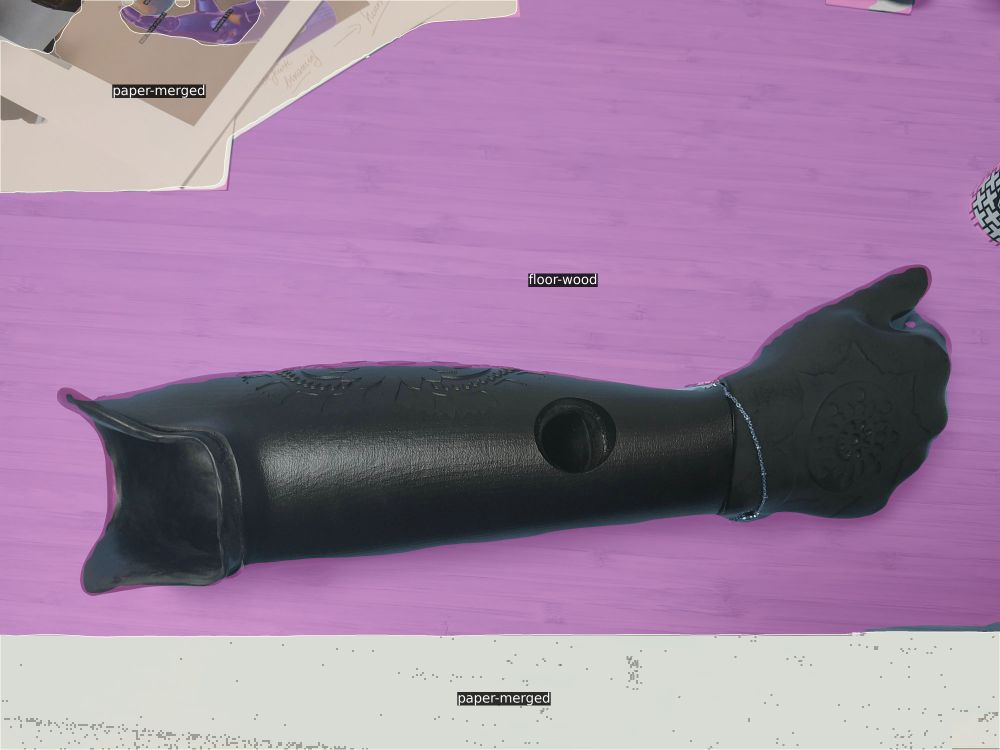
Societal ignorance
One participant noted that the above misrepresentations are not just a technical problem, but also a wider societal issue. While he felt social acceptance of prosthetics has increased tremendously over the last decades, he still finds a lot of ignorance around it as well.
For instance, people in public often don’t know how to react to and name prosthetics. It is not unexpected then, that an image recognition system build from that society can be ignorant as well.
Diversity of prosthetics
Of course, there is also a wide variety of prosthetics available. For one, they can function as different parts of the body, such as the lower limbs, upper limbs, only a hand, or only a finger.
Secondly, the appearance of the prosthetic can be very different as well. Some people choose to have the metal or plastic of the prosthetic visible, while others prefer to put a sleeve with more skin-like textures and colors on top.
Thirdly, prosthetics can be decorated, for instance with tattoos. This again results in a very different visual pattern that image recognition systems would need to be able to recognize.
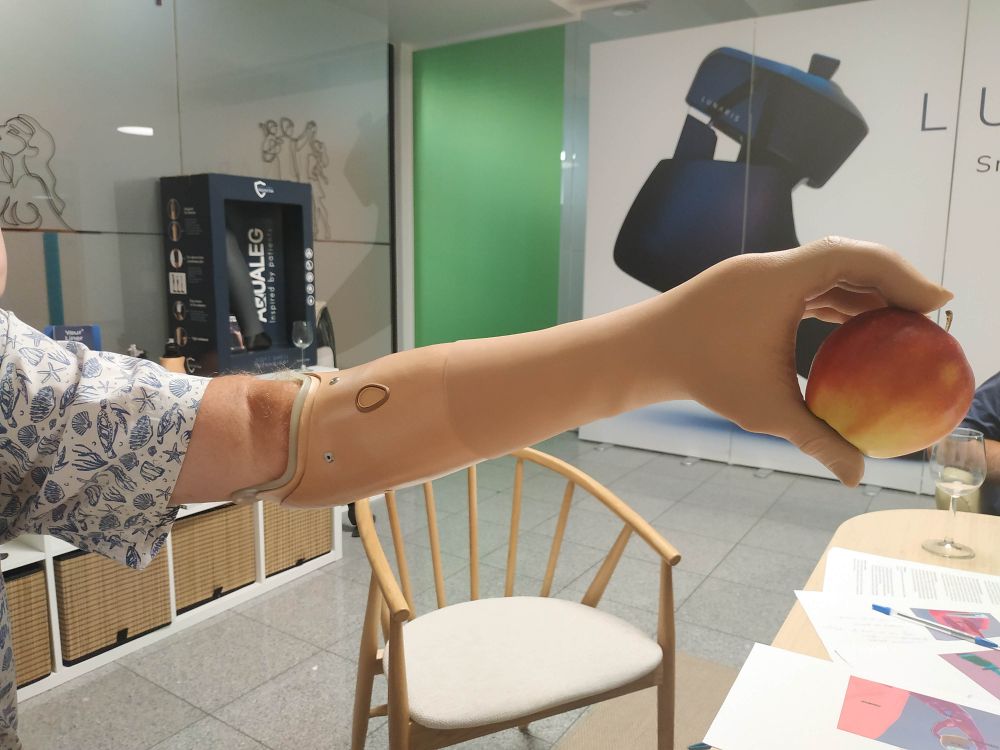
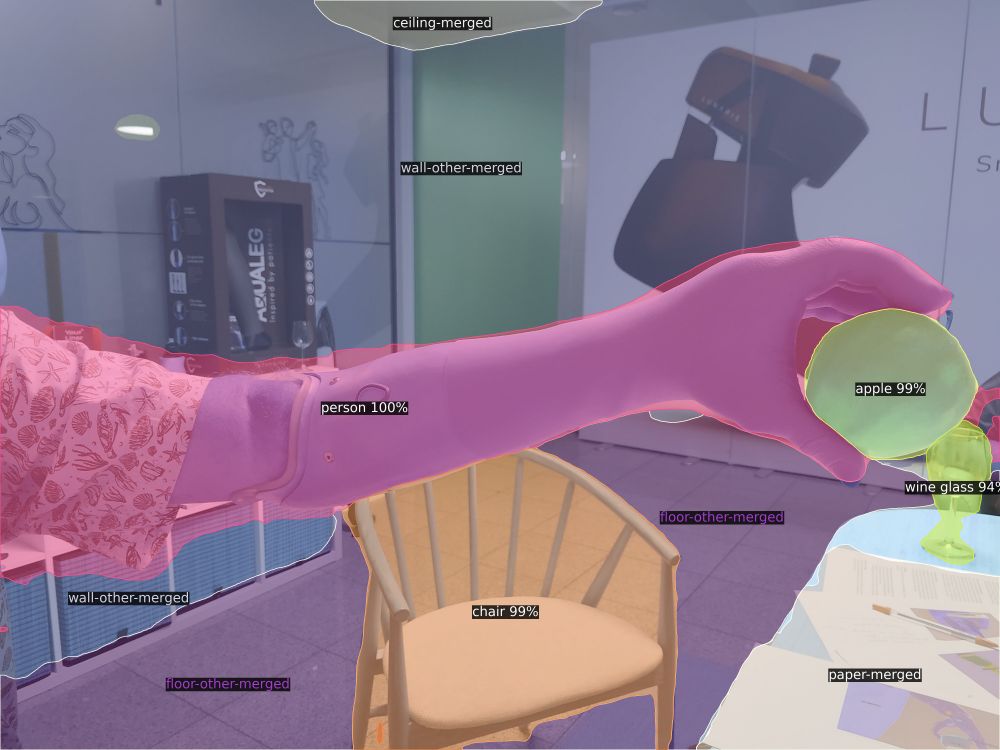
The difference in prosthetics can have a significant impact on the results. For example, the arm prosthetic with a Caucasian skin-like color in the image above, is recognized as part of the person, giving very different results than the similar image above where an arm prosthetic was labeled “chair”.
Impact of mislabeling
For the participants, the mislabeling was often seen as weird and incomprehensible, yet not offensive per se. The topic of “acceptance” became very prominent here. All the participants had lived with the prosthetic for quite some time and were very comfortable with it.
“Someone is going to need to take a lot more pictures.”
– A participant when seeing his prosthetic labeled as “toilet”.
For example, in the picture below, the system registers a participant’s leg prosthetic as “toilet”. However, the response of the participant simply was: “Someone is going to need to take a lot more pictures” (in extension of having explained how most image recognition systems are build). This indicates that he could laugh off the mistake of the system without being affected by it too much.
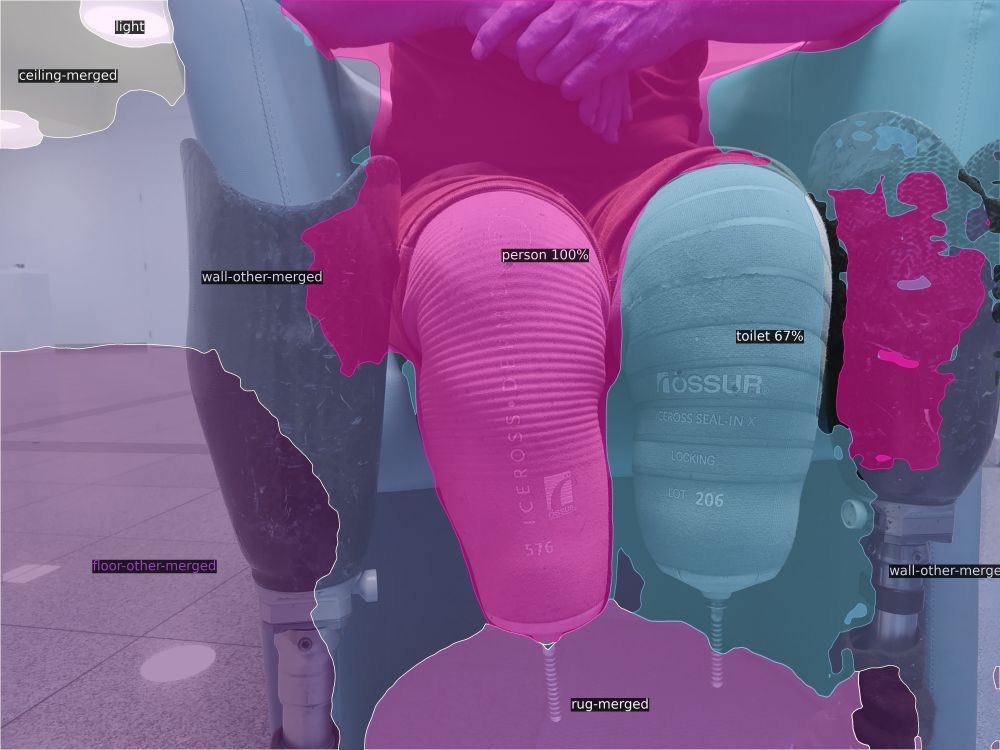
Nevertheless, the participating coordinator of the center noted she often has people who just received a prosthetic (e.g. after an accident). These people have not yet been able to make full sense of the fact they wear a prosthetic and haven’t become comfortable with it yet.
She noted that for these people, the chance is very high that results such as “chair” or “toilet” would be very confrontational. When an image recognition system would use such labels, the process of acceptance would become even harder.
The difference between participants
While the above general themes emerged from this work, it should be noted that people wearing prosthetics is not a uniform group in itself. As such, people wearing prosthetics do not necessarily hold the same views of how to be represented by image recognition systems.
For example, while one participant was telling that though he was quite comfortable with his prosthetic, he hates the fact that he needs to explain his story again and again to new people he meets. Conversely, another person replied that he quite likes having conversations with people, including about his prosthetics. He sees it as part of his life story and identity, and therefore likes to talk about it.
What data scientists can learn.
The work presented in this article puts an initial light on the problematic manner state-of-the-art image recognition systems interpret people wearing prosthetics. In this concluding section, we provide three important recommendations for data scientists working on these systems.
The need for diverse data science teams
As described in the previous section, the discussed misrepresentations can be seen as stemming from a society not yet fully embracing of the concept and reality of prosthetics.
While this resonates with the call for more diverse data science teams within the AI community, it’s hard to deny that even those teams are formed from the fabric of the same society that hasn’t embraced prosthetics fully yet, which makes the chances high the resulting system will not have either.
Perhaps this issue is not solely one of knowledge, but more so one of empathy and familiarity.
And so, perhaps this issue is not solely one of knowledge, but more so one of empathy and familiarity. For instance, by reading this article you’ll gain some knowledge on the development choices you make as a data scientist, but it is only by actually becoming intimately familiar with the reality of wearing a prosthetic that you can add depth and confidence to these choices.
Nevertheless, team members that have that intimate familiarity with prosthetics can bring a significant advantage to data science teams.
The non-uniformity of people wearing prosthetics
It is easy to think that AI systems need to be build in one specific way so they suite everybody. Unfortunately, that’s not how it works. People see things in different ways and prefer things in ways other people might not.
Data science teams should therefor preferably abandon the idea that they should build more inclusively for a uniform group of people wearing prosthetics. While the general tenet of this goal is virtuous, it misses the nuance that people within this group have very different preferences.
It is therefore recommended that when building AI systems, thought should be given to whom exactly the system should be build for, even within the group of prosthetics wearers. Furthermore, people from this group should be included in the development process.
The impact of misrepresenting prosthetics
Data science teams need to be aware of the impact wrong labels can have on people wearing prosthetics. While some people can put absurd labels into perspective, others might not yet be able to do this. For the latter group, a denigrating misrepresentation might cause significant emotional stress.
Furthermore, it is still unclear what the practical impact of such misrepresentations will have as image recognition systems become more and more integrated into society, products, and services.
Thank you
I’d like to extend a warm thank you to the Amputee Care Center by Spronken and the participants for taking the time and putting in the effort to participate in this study.
*For this study, the state-of-the-art image segmentation model Mask2Former was used.
An adapted version of this article has also been published by Knowledge Centre Data & Society.