Creating Value in Multi-Ethnic Food Detection Systems
Context
Being able to detect food in images with Artificial Intelligence opens up a lot of potential value for food delivery services and social network services.
However, the enormous variety of foods across countries and cultures makes it difficult for data scientists to identify what users of these services find valuable in specific contexts.
I therefore set out to gain exploratory insights on where value can be created when tailoring a major image recognition framework* to a multi-ethnic production context of food in 13 countries across four continents**.
Rice with chicken chili curry from Myanmar Sirene Po Shopski from Bulgaria
Process
I invited people from the 13 different countries in question to share a digital meal with me over video chat. I requested them to prepare a meal they would otherwise also prepare.
During our meal, the participants told me about their meal, sent me pictures of their meal, and described the foods of their country. Through secondary research, I gained additional information on food in each country.
Finally, I ran the collected images from each participants through the image recognition frameworks and analyzed the results.
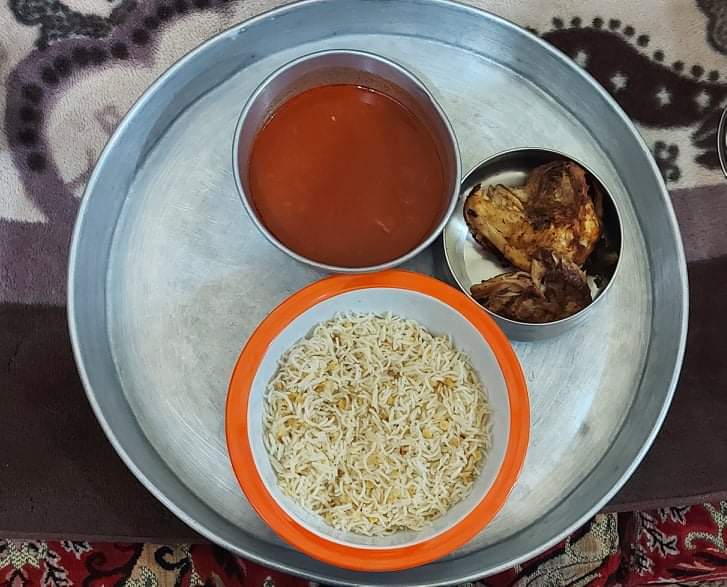
Insights
A number of insights on adding value to multi-ethnic food recognition systems were gained. I encourage data scientists working on these particular systems to:
Create more specific and relevant labels
A lot of value can be added by providing more relevant labels for the current production context. The most impact would be gained by labeling and training for: spring rolls, bagels, bottle of wine, Cuy, Kaydos, crab cakes, among others.
Address visual misrepresentations
Though completely different dishes, some look visually so similar they confuse the current recognition systems. A lot of user value is to be gained by improving performance between visually similar dishes, for instance:
- Spring rolls should be distinguishable from sausages.
- Rice, Kaydos and Crab cakes should be distinguishable from ice cream.
- Sliced melon should be distinguishable from bananas.
- Rice should be distinguishable from oatmeal.
- Cuy should be distinguishable from pizza.
- And more.
Address cultural misrepresentations
On the other hand, some dishes are similar, but come from culturally sensitive contexts. My research indicates that negative attitudes could arise when predictions fail to acknowledge different cultural contexts. For instance:
- A Thali (Indian serving plate) in an image should not skew Yemeni food results towards Indian food results.
- A roti should be distinguishable from a tortilla or pita.
- Frittata should be distinguishable from a pizza.
- Minestrone should be distinguishable from a curry.
- Chopsticks should be as recognizable as forks and spoons.
- And more.
Do not harm people of certain religions or with certain diets with labels of meat
In many contexts, meat is a sensitive topic. Some people avoid certain meats because of religions beliefs, others because of medical conditions, and others still because of moral beliefs. Presenting faulty predictions about meat towards these audiences could be seriously detrimental to the value image recognition systems can offer.
Based on the current investigation, we therefore recommend improving the following predictions:
- Cuy should not be recognized as duck and chicken meat.
- Carrot cake should not be recognized as steak, beef, or meat loaf.
- Mock meat should not be recognized as real meat.
- Chicken should not be recognized a s beef.
- And more.
Address sensitive predictions
Alcohol and weapons are two sensitive topics when presenting predictions in multi-ethnic production contexts. Concerning alcohol, data scientists would do well to prioritize the performance of predictions on food items containing alcohol (such as a bottle of wine),. The current systems often performed poorly in this respect.
In multiple instances, dinner knifes were labelled as weapons. Though less critical, data scientists are invited to explore the degree to which their food detection systems need to label weaponry.
Recognize the limits of image recognition systems
Image recognition technology is very exciting, but data scientists who want to create value for users in production need to make sure to recognize the technology’s limits. For instance:
- Can a system actually detect if a waffle has Kaya and Margarine on it based only on an image without further context?
- Can a system actually detect if a meal is gluten or sugar free simply based on an image without further context?
- And more.
Notes
* This exploration included an analysis of Microsoft Azure, Google Vision, Amazon Rekognition and IBM Watson
**Belgium, Myanmar, Vietnam, Malaysia, the Philippines, Canada, the US, Yemen, Germany, England, Singapore, Bulgaria, and Ecuador.